A right whale and her right calf. Source: NOAA |
Wednesday, November 25, 2015
Talking Data Podcast ponders analytical algorithms to help save whales
Tuesday, November 17, 2015
Architectures from different views
rdf..its all about the triples.. you nurse it an rehearse it. the idea of a sentence.
take a bunch of different types of data from different sources .. put them all together in triples
on another level you layer it on a physical data infrastructure architecture
Monday, November 9, 2015
Tensor
Let’s look inside a Learning Machine - Google Tensor
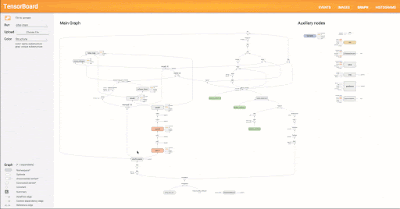
https://blogger.googleusercontent.com/img/b/R29vZ2xl/AVvXsEi-5K0GDYQsrw_oRhC_2hQ0lOp5xC0n1GRxtnpViaFNmriieL3FWkHQRf9QJS_5W7ZMPilxA-MxibJO4A9dygaRSqj0x15klMEstjZ7JDbVtg282JnG8IRd4wTTBEfS828paM2UEQi-Vk4/s1600/cifar10_2.gif
http://www.tensorflow.org/
http://googleresearch.blogspot.com/2015/11/tensorflow-googles-latest-machine_9.html
http://googleresearch.blogspot.com/2015/11/computer-respond-to-this-email.html
https://www.youtube.com/watch?v=46Jzu-xWIBk
https://www.youtube.com/watch?v=gY9DewL6Dqk
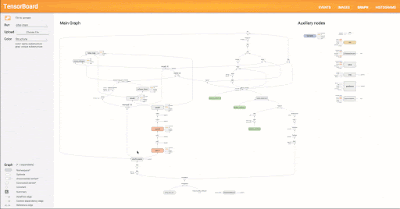
https://blogger.googleusercontent.com/img/b/R29vZ2xl/AVvXsEi-5K0GDYQsrw_oRhC_2hQ0lOp5xC0n1GRxtnpViaFNmriieL3FWkHQRf9QJS_5W7ZMPilxA-MxibJO4A9dygaRSqj0x15klMEstjZ7JDbVtg282JnG8IRd4wTTBEfS828paM2UEQi-Vk4/s1600/cifar10_2.gif
http://www.tensorflow.org/
http://googleresearch.blogspot.com/2015/11/tensorflow-googles-latest-machine_9.html
http://googleresearch.blogspot.com/2015/11/computer-respond-to-this-email.html
https://www.youtube.com/watch?v=46Jzu-xWIBk
https://www.youtube.com/watch?v=gY9DewL6Dqk
Friday, November 6, 2015
Machine learning for better medical income on TAP
Michael Draugelis, is chief data scientist, The University of Pennsylvania, Penn Medicine. He came to this gig in a way roundabout. You see, his wife went into shock while giving birth to their child, Chubsy Ubsy. Mother and child are doing well, but the experience made Draugelis wonder. That's because of his background in US Missile Defense Agency, where they did a lot of work about forecasting clues to impending events. He appeared at Strata + East to discuss all this.
When he got to Penn Med he set out to focus on Sepsis, an unfortunately leading cause of death for people who go to the hospital to fix something else. His data scientist efforts revolve around something called Penn Signals. "A nerd wonderland," says he. The point is to use evidence-based computing to see who is really really at risk. Right now!
Now, of course, in reality, he is doing what all the machine learning people are doing:
We have some early proof of concepts that have created new signals..that we are feeding back into our algorithms.
What is next?
I hope to move on from prediction to auto reasoning.
That is where his work with Intel comes in.
The company, which would like to fight disease just as much as it would like to sell chips that go into servers on Web farms, has concluded that big data development, where egg headed data scientists create models in Python etc., that must be thrown over the wall, and rejiggered in Java by 'real' developers, and then sent back to fix, and then back over the wall again etc., was just going to take too much time. Why not more of a cloud development paradigm? Based on open source? Tested by Intel and the like of Penn? It's called TAP, for Trusted Analytics Platform.
Says our man Draugelis:
My data scientists need an environment that they can build quickly, select their analytic tools at scale, and a platform that can support it. We have been excited to work with Intel to explore this new open source project called TAP.
As a colleague said, or more precisely asked: " What was the last big software announcement Intel made? And what came of it? And yes the answer is The Intel Hadoop Distribution and what came of it was a ceding of the work to Cloudera.So to coin a phrase: Time will tell. - Jack Vaughan
For more
Go to github to find out what it would be like to be a cloud developer these days.
Listen to a podcast where we talk with Intel's Vin Sharma about TAP
You see that embedded video above, this here is the linke to it, like actually.
When he got to Penn Med he set out to focus on Sepsis, an unfortunately leading cause of death for people who go to the hospital to fix something else. His data scientist efforts revolve around something called Penn Signals. "A nerd wonderland," says he. The point is to use evidence-based computing to see who is really really at risk. Right now!
Now, of course, in reality, he is doing what all the machine learning people are doing:
We have some early proof of concepts that have created new signals..that we are feeding back into our algorithms.
What is next?
I hope to move on from prediction to auto reasoning.
That is where his work with Intel comes in.
The company, which would like to fight disease just as much as it would like to sell chips that go into servers on Web farms, has concluded that big data development, where egg headed data scientists create models in Python etc., that must be thrown over the wall, and rejiggered in Java by 'real' developers, and then sent back to fix, and then back over the wall again etc., was just going to take too much time. Why not more of a cloud development paradigm? Based on open source? Tested by Intel and the like of Penn? It's called TAP, for Trusted Analytics Platform.
Says our man Draugelis:
My data scientists need an environment that they can build quickly, select their analytic tools at scale, and a platform that can support it. We have been excited to work with Intel to explore this new open source project called TAP.
As a colleague said, or more precisely asked: " What was the last big software announcement Intel made? And what came of it? And yes the answer is The Intel Hadoop Distribution and what came of it was a ceding of the work to Cloudera.So to coin a phrase: Time will tell. - Jack Vaughan
For more
Go to github to find out what it would be like to be a cloud developer these days.
Listen to a podcast where we talk with Intel's Vin Sharma about TAP
You see that embedded video above, this here is the linke to it, like actually.
Subscribe to:
Posts (Atom)